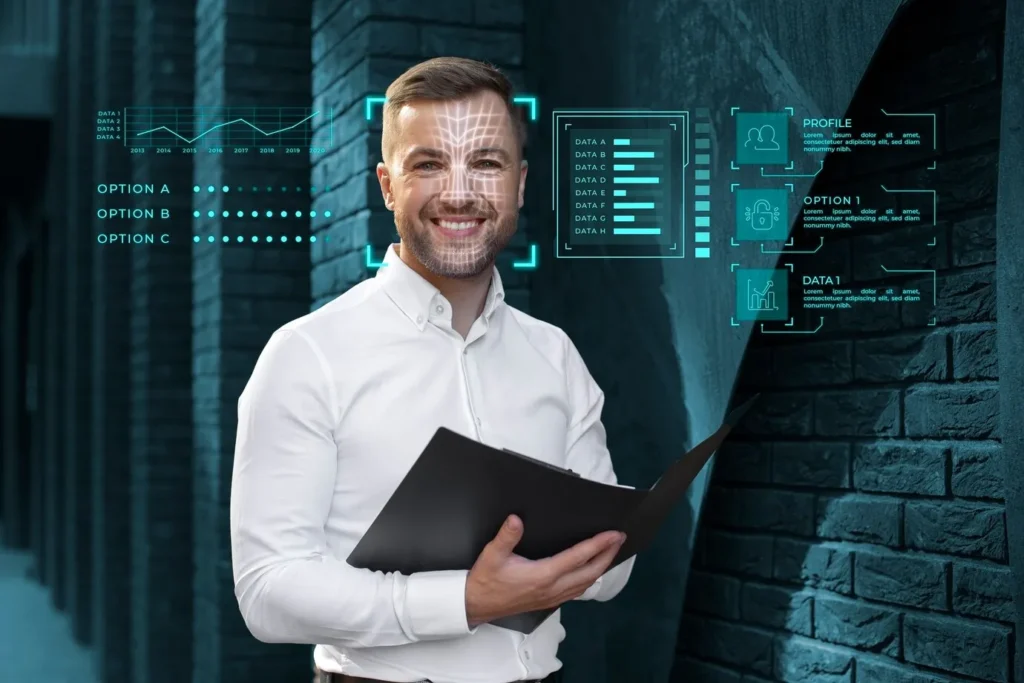
We all know how Data engineering services are essential when it comes to the success of artificial intelligence models. Businesses around the world are going to work on data-driven decision-making and this is where the role of data engineering becomes essential.
So, if you are thinking about how data engineering is going to support artificial intelligence models then you have certainly landed on the right page. We are here to help you understand every aspect about it in detail. Read on.
Data Engineering: All You Must Know About It
Data engineering is a set of operations meant to get data ready for analysis and application in artificial intelligence. Several important elements of this procedure are:
Data Collection
The data engineering method begins with data gathering. It means collecting data from many different places, including databases, APIs, and real-time streams. The aim is to gather a varied dataset that can properly train artificial intelligence systems.
Data Cleansing
Data typically needs cleaning to guarantee its quality and dependability after collection. Data cleansing is the process of finding and fixing dataset inaccuracies or discrepancies. Because artificial intelligence models depend on high-quality data to generate precise forecasts, this stage is absolutely vital.
Data Transformation
Data transformation is the process of turning raw data into a form appropriate for analysis. This could mean standardizing numbers, data aggregation, or generating new characteristics improving the use of the dataset. Effective transformation guarantees appropriate data interpretation by the artificial intelligence models.
Data Storage
Large amounts of data must be managed effectively using data storage systems. This covers choosing suitable cloud storage options or databases capable of handling both unstructured and structured data. For artificial intelligence applications and EDI Services, the speed and accessibility of the data are affected by the choice of storage.
The Role of Data Engineering in AI Development
The relationship between data engineering and AI development is symbiotic. While data engineering lays the groundwork for AI initiatives, advancements in AI also enhance data engineering processes.
Enabling AI Model Training
Data engineers play a vital role in preparing datasets that are used to train AI models. They ensure that the datasets are comprehensive, relevant, and free from biases that could skew results. By providing high-quality training data, data engineers enable AI development companies to create more accurate and reliable models.
Automation of Data Processes
The integration of AI into data engineering processes has led to increased automation. For instance, machine learning algorithms can automate routine tasks such as data ingestion and cleansing. This not only saves time but also reduces the potential for human error in these processes.
Enhancing Data Quality
AI technologies can assist in monitoring and improving data quality continuously. By analyzing metadata and identifying patterns within datasets, AI can help data engineers pinpoint issues related to data integrity or completeness. This proactive approach ensures that the datasets remain reliable over time.
Real-World Applications of Data Engineering in AI
The collaboration between data engineering and AI has resulted in numerous innovative applications across various industries.
Autonomous Vehicles
In the automotive industry, autonomous vehicles rely heavily on vast amounts of sensor data to navigate safely. Data engineers design systems that collect and process this information in real-time, enabling AI algorithms to make split-second decisions based on current conditions.
Personalized Recommendations
E-commerce platforms utilize sophisticated recommendation systems powered by AI. Data engineers create pipelines that aggregate user behavior data, product information, and transaction history to deliver personalized recommendations to customers. This enhances user experience and drives sales.
Healthcare Analytics
In healthcare, predictive analytics powered by AI can lead to better patient outcomes. Data engineers develop systems that integrate patient records, clinical trials, and research studies into cohesive datasets. These datasets allow healthcare providers to identify trends and make informed decisions regarding patient care.
Challenges Faced by Data Engineers in Supporting AI Models
While the integration of data engineering and AI presents numerous benefits, it also comes with challenges that need addressing.
Volume of Data
The sheer volume of data generated today poses a significant challenge for data engineers. Managing large datasets requires robust infrastructure capable of handling real-time processing without compromising performance.
Data Privacy Regulations
With stringent regulations surrounding data privacy such as GDPR and CCPA, ensuring compliance while managing sensitive information is paramount for data engineers. They must implement measures to anonymize or secure personal information without sacrificing the quality of the datasets used for training AI models.
Skill Gaps
As technology evolves rapidly, there is often a skills gap among existing professionals in both fields. Continuous education and training are necessary to equip data engineers with the knowledge needed to leverage advanced tools and techniques effectively.
Data Engineering for AI: What Lies Ahead
The landscape of data engineering is continuously evolving alongside advancements in AI technology. Several trends are shaping its future:
Increased Use of Generative AI
By automating difficult procedures like schema development and feature engineering, generative AI is transforming how data engineering activities are carried out. These technologies will eventually simplify processes inside companies as they develop.
Focus on Real-Time Analytics
Organizations are wanting real-time insights more and more from their data. Consequently, more focus will be placed on creating systems able to process streaming data effectively while preserving analytical accuracy.
Enhanced Collaboration Between Teams
As companies realize the need of cross-functional teams in driving innovation inside their AI projects, the cooperation between data engineers and other stakeholders including data scientists will become more obvious.
Final Thoughts
Looking ahead, it is obvious that innovative development in many sectors will be driven by efficient cooperation between data engineering and artificial intelligence. While encouraging a culture of lifelong learning among their workers, organizations have to give strong infrastructure first importance. Moreover, businesses will require knowledgeable people who grasp both fields as they investigate enterprise AI chatbot development since their investments will produce the best results in an ever more complicated environment driven by technology.